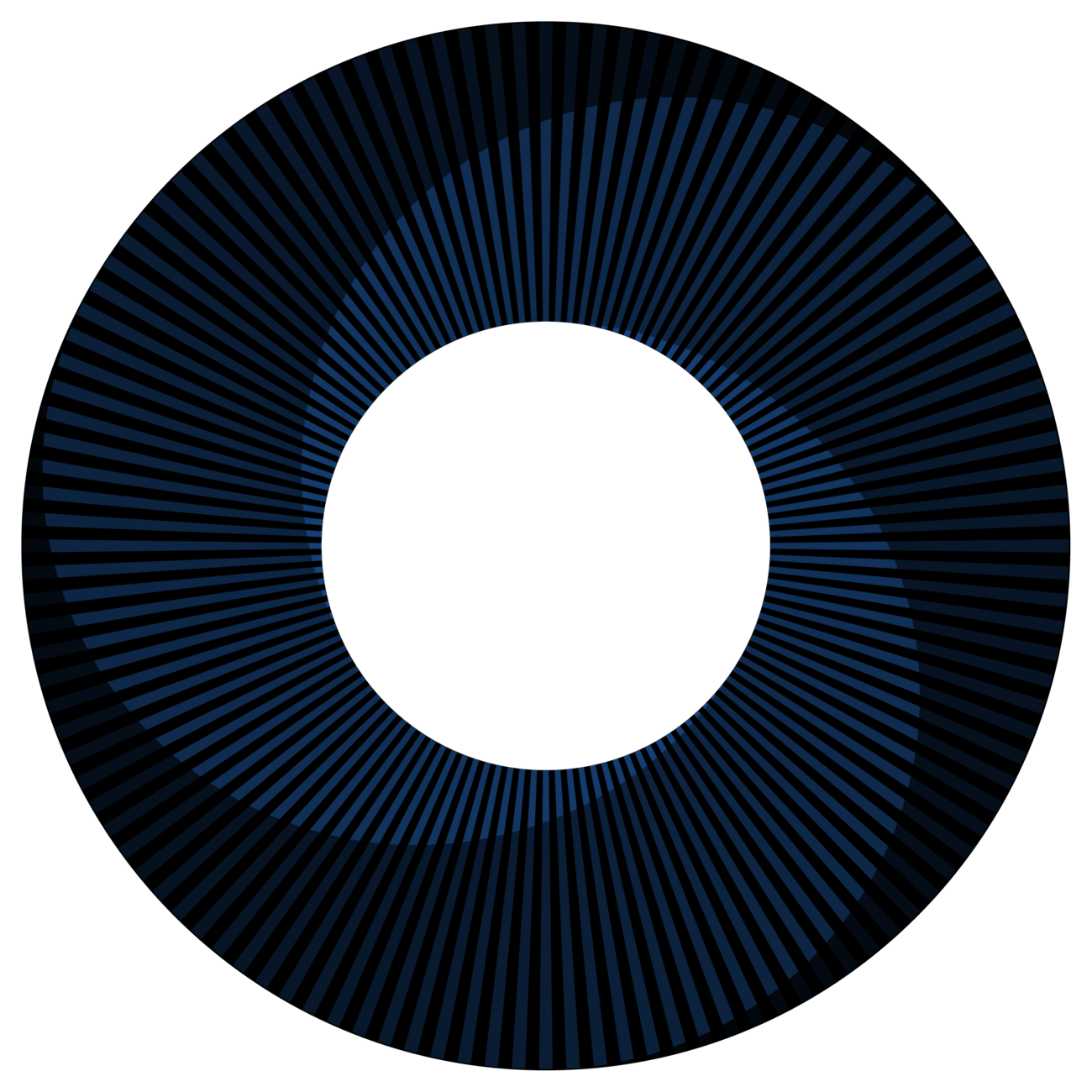
November 28, 2023
November 2023 - Leveraging TAR beyond its most common usage to reduce cost, shorten timelines and improve outcomes
For nearly two decades, technology-assisted review has been used in e-discovery matters to reduce costs, increase efficiencies and speed up manual review of documents for the purposes of production. The technology most commonly behind current TAR models is supervised machine learning, a subset of AI.
Supervised machine learning employs labeled datasets to train algorithms to classify data or predict outcomes accurately. There are currently two main approaches to TAR using supervised machine learning:
TAR 1.0 (Predictive coding): The OG of TAR, predictive coding involves loading a seed set of documents into the system to train the predictive algorithm. The quality of the results will depend on the quality of this original seed set, which can only be altered by adding subsequent sample sets with updated coding to retrain the machine.
TAR 2.0 (Continuous active learning or CAL): Developed more recently, CAL does not require a seed set. The algorithm automatically learns from the reviewers’ decisions and starts feeding the review team what it determines are the most relevant documents based on the purpose of the model. The system gains intelligence as it receives further inputs (decisions) from human reviewers.
To date, TAR models have been implemented to classify documents based on binary decisions – responsive/not responsive or relevant/not relevant. But the innovation and improvements in concepts used in TAR methodologies today, such as supervised machine learning, AI and sophisticated algorithms, have expanded the utility of TAR to other workflows that are relatively common in the e-discovery review life cycle.
A few examples include:
- Snapshot Analysis of Your Data and Its Richness: Getting a quick, high-level view of the document set gives lawyers an early understanding of the key issues in the case, enabling them to craft a better review strategy. It also helps to structure the case at the outset and identify parties to depose, defenses to assert and other key metrics important to the matter. Finally, leveraging TAR in this manner can help to inform decisions on whether it makes sense to settle a matter quickly or proceed with litigation.
- Key Document Identification: Instead of your TAR model being trained to focus on responsiveness for purposes of production, you can create and train your model to focus on identifying key documents – either helpful or harmful – early in the discovery process.
- Issue Identification: Just as it can help identify key documents, TAR models can be utilized to get a clear picture of what documents in your dataset are related to a specific or important issue.
- Compliance Monitoring/Identification of Fraudulent Behavior: While it takes investment and foresight, TAR can be utilized in a programmatic way to ensure that employees are complying with relevant laws, regulations or company standards as well as to uncover any fraudulent or nefarious activities performed by employees. Using TAR for compliance monitoring and/or identification of bad actors/activities may be particularly useful for companies in highly regulated industries.
The data landscape is always changing, with today’s technologies generating large amounts of complex information that must be analyzed to understand the totality of a data picture. But current TAR models are not built for these new types of data. So for TAR to continue being useful in tackling the above instances and others in the future, it will need investment in continuous improvement to include more advanced AI technology with trained experts and linguistics to help wield them.
QuisLex vice president, legal solutions and strategic growth, Robert Coppola shared his insight with a deeper dive on this topic in the recent article Expanding the Use of TAR Beyond Its Common Utility, published by ILTA Peer to Peer magazine.